The phenomenon of AI hallucination has sparked significant discussions in the world of artificial intelligence, particularly with the rise of generative AI and large language models (LLMs). While AI systems are becoming increasingly sophisticated, their tendency to produce outputs that are either fabricated, nonsensical, or entirely incorrect poses challenges for developers, users, and industries reliant on AI-generated content.
This comprehensive guide explores the causes, examples, implications, and possible solutions to Artificial Intelligence hallucination, shedding light on this complex issue.
Introduction to AI Hallucination
The concept of AI hallucination refers to instances where artificial intelligence systems, particularly large language models (LLMs), produce information that is inaccurate, fabricated, or entirely made up. Unlike human errors, which can often be tied to misunderstanding or lack of knowledge, AI hallucinations result from how these systems generate content.
What is AI Hallucination?
AI hallucination occurs when an AI system generates content that appears plausible but is factually incorrect or nonsensical. These errors can manifest in several forms, including:
- Providing incorrect answers to factual questions.
- Generating fictitious citations or references.
- Producing creative but misleading outputs.
Why Does AI Hallucination Happen?
- Predictive Nature of AI: Generative AI models, like GPT or other LLMs, predict the next word or sequence based on their training data. This process can sometimes result in coherent but incorrect outputs.
- Training Limitations: AI systems rely on vast datasets for training. If these datasets contain biases, inaccuracies, or gaps, hallucinations can occur.
- Complex Queries: When faced with complex or ambiguous prompts, AI systems might fabricate information to fill the gaps.
Understanding the root causes of Artificial Intelligence hallucination is crucial for developing strategies to minimize its occurrence.
Examples of AI Hallucination
Examples of this can be found across various applications of artificial intelligence, from chatbots to image generators.
Common Cases
- False Citations: AI models often generate academic references or article citations that do not exist.
- Misinformation: Providing incorrect answers to factual questions, such as dates or historical events.
- Fabricated Scenarios: Producing fictional scenarios in response to prompts that require factual accuracy.
Real-World Implications
The impact of AI hallucination extends beyond mere inconvenience:
- Legal Consequences: Incorrect legal advice generated by AI can lead to severe repercussions.
- Misinformation Spread: AI-generated falsehoods can perpetuate myths or fake news.
- Trust Erosion: Repeated hallucinations may lead users to distrust AI systems altogether.
These examples highlight the importance of addressing AI hallucination to ensure the reliability of AI systems.
The Role of Large Language Models in AI Hallucination
Large language models (LLMs), the backbone of many generative AI systems, play a significant role in the prevalence of this.
How LLMs Work
LLMs, like GPT, are trained on extensive datasets comprising text from the internet, books, and other sources. They generate content by predicting the next word in a sequence, based on patterns learned during training.
- Strengths: They excel in generating coherent, contextually appropriate content.
- Weaknesses: Their reliance on probabilistic methods can lead to plausible-sounding but incorrect outputs.
Why LLMs Hallucinate
- Data Quality Issues: Training data often contains errors or biases, which the AI inadvertently learns.
- Ambiguity Handling: When faced with ambiguous or incomplete prompts, LLMs may fabricate answers rather than admit uncertainty.
- Overfitting: AI systems sometimes overgeneralize patterns from training data, leading to unrealistic outputs.
Addressing these issues requires refining both the training data and the algorithms underpinning LLMs.
Preventing AI Hallucination
Minimizing AI hallucination involves a combination of technical, procedural, and user-focused strategies.
Techniques to Reduce Hallucination
- Data Curation: Ensuring high-quality, unbiased training data minimizes errors learned during training.
- Fine-Tuning Models: Adapting AI models for specific industries or tasks can reduce inaccuracies.
- Feedback Loops: Incorporating user feedback allows AI systems to learn from their mistakes.
User Best Practices
- Verify AI Outputs: Users should cross-check AI-generated information with reliable sources.
- Provide Clear Prompts: Ambiguity in user prompts can increase the likelihood of hallucinations.
While AI hallucination cannot be eliminated entirely, these strategies can significantly reduce its occurrence.
Ethical Implications of AI Hallucination
The ethical ramifications of AI – hallucination extend beyond technical considerations, affecting trust, accountability, and societal impact.
Trust in AI
When AI hallucination occurs frequently, users may lose trust in AI systems, limiting their adoption in critical fields like healthcare or law.
Accountability Issues
Determining accountability for AI-generated errors is a challenge:
- Developers: Responsible for building and training the AI system.
- Users: Responsible for interpreting and acting on AI outputs.
Addressing Ethical Concerns
Ethical AI development requires transparency, robust testing, and mechanisms to mitigate harm caused by hallucinations.
Industries Impacted by AI Hallucination
The consequences of AI – hallucination are particularly evident in industries that rely heavily on accurate information.
Healthcare
Errors in medical advice or diagnoses generated by AI systems can lead to dire consequences for patients.
Legal Sector
False information generated by AI in legal contexts can result in incorrect decisions or even miscarriages of justice.
Education
AI-generated misinformation in educational tools can mislead students and educators.
Industries must adopt stringent measures to address AI hallucination and ensure the reliability of AI systems.
Future Trends in AI Hallucination
As AI technology evolves, new approaches to mitigating AI hallucination are emerging.
Advances in AI Training
- Reinforcement Learning with Feedback: Training AI systems to learn from user corrections.
- Hybrid Models: Combining AI-generated insights with human oversight to ensure accuracy.
The Role of Regulation
Governments and organizations are beginning to establish standards and regulations to address AI-related issues, including hallucination.
By staying informed about these trends, stakeholders can better navigate the challenges of AI hallucination.
How Users Can Adapt to AI Hallucination
Users of AI systems play a critical role in managing and mitigating the effects of Artificial Intelligence hallucination.
Tips for Users
- Educate Yourself: Understand the limitations and capabilities of AI systems.
- Cross-Verify Information: Never rely solely on AI-generated outputs without verification.
- Report Issues: Providing feedback to AI developers helps improve system performance.
Conclusion
Artificial Intelligence hallucination represents a significant challenge in the development and deployment of artificial intelligence. By understanding its causes, consequences, and potential solutions, both developers and users can work together to reduce its impact. While hallucinations are unlikely to be eradicated entirely, ongoing advancements in AI technology, coupled with responsible usage, promise to make AI systems more reliable and trustworthy in the future.
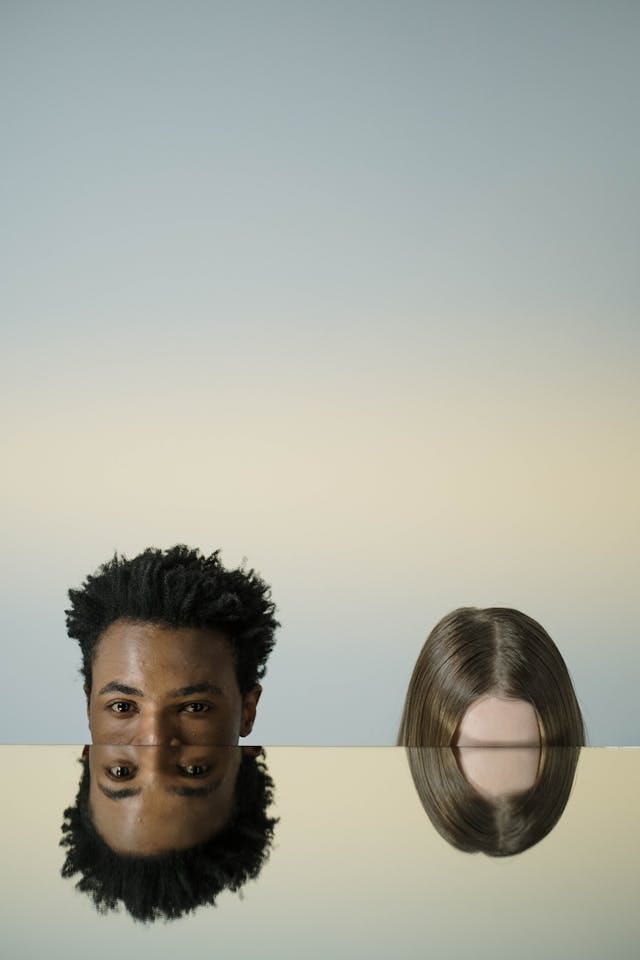