In recent years, generative artificial intelligence (AI) has emerged as a transformative force across various sectors, including healthcare. Among its many applications, generative AI is proving particularly promising in the realm of mental health assessment. As society grapples with increasing mental health challenges, understanding how generative AI can unlock insights into mental well-being has become essential. This article delves into the fundamental concepts of generative AI, explores its applications in mental health assessment, and examines the potential benefits and challenges associated with its use.
By leveraging the capabilities of generative AI, mental health professionals can enhance their understanding of patients’ needs and provide more tailored interventions. This article will cover the mechanics of generative AI, its integration into mental health practices, and future implications for the field.
What is Generative AI?
Generative AI refers to a class of artificial intelligence that can generate new content or data based on patterns and information it has learned from existing data. Unlike traditional AI models, which focus on classification or prediction, generative AI models create new outputs—such as text, images, or audio—by learning from extensive datasets.
Key Components of Generative AI
Machine Learning Algorithms: Generative AI leverages various machine learning algorithms, including neural networks, to understand patterns and relationships within data. For more information on machine learning, visit IBM.
Data Input: The effectiveness of generative AI heavily relies on the quality and diversity of the input data. Larger datasets often lead to more accurate and nuanced outputs.
Model Training: Generative models undergo training to learn the underlying structures of the data. This training process is critical for generating coherent and relevant content.
Generative Models: Some well-known types of generative models include Generative Adversarial Networks (GANs), Variational Autoencoders (VAEs), and Transformer-based models like GPT-3. For a deeper understanding of GANs, refer to this Research Paper.
The Role of Generative AI in Mental Health Assessment
1. Data Analysis and Interpretation
Generative AI can assist mental health professionals by analyzing vast amounts of patient data, including text from therapy sessions, surveys, and social media interactions. By identifying patterns in this data, AI can help clinicians gain insights into patients’ emotional states, behaviors, and potential mental health issues.
For instance, algorithms can analyze language use in patients’ communications to detect changes that may indicate worsening depression or anxiety. This can lead to earlier interventions and more tailored treatment plans. Learn more about data analysis in healthcare from Harvard Business Review.
2. Predictive Analytics
Generative AI models can be trained to predict mental health trends based on historical data. By analyzing past patient outcomes, these models can forecast future mental health challenges, allowing healthcare providers to proactively address issues before they escalate.
Predictive analytics can also help in identifying individuals at higher risk for mental health disorders based on various factors, such as demographics, medical history, and social determinants of health. Explore more on predictive analytics in healthcare at McKinsey.
3. Personalized Treatment Plans
Generative AI can contribute to creating personalized treatment plans by analyzing individual patient data and comparing it with a broad dataset. This approach can help therapists tailor interventions to meet each patient’s unique needs and preferences, ultimately improving treatment efficacy.
For example, generative AI can suggest specific therapeutic techniques or medication adjustments based on similar cases from the past, enhancing the likelihood of positive patient outcomes. The National Institutes of Health provides further information on personalized medicine in mental health.
4. Virtual Therapy and Support
AI-driven chatbots and virtual therapists are increasingly being used to provide mental health support. These generative AI tools can engage users in conversation, offer coping strategies, and monitor mood changes over time. While they do not replace human therapists, they can supplement traditional therapy by providing immediate support to those in need.
To understand the implications of virtual therapy, you can read about it on Psychology Today.
5. Enhancing Diagnostic Accuracy
Generative AI has the potential to enhance diagnostic accuracy by analyzing patient data more comprehensively than traditional methods. By examining a wide range of symptoms and contextual factors, AI can assist clinicians in making more accurate diagnoses and identifying comorbid conditions.
For instance, generative AI can help detect subtle signs of mental health issues that may be overlooked during a standard assessment, leading to more informed clinical decisions. For additional insights on diagnostic accuracy in mental health, refer to American Psychological Association.
Benefits of Using Generative AI in Mental Health Assessment
1. Improved Access to Care
Generative AI can help bridge the gap in mental health care access by providing scalable solutions. Virtual therapists and chatbots can reach individuals in remote areas or those with limited access to traditional mental health services. This increased accessibility can lead to earlier identification and intervention for mental health issues.
Explore how AI can improve access to care at World Health Organization.
2. Enhanced Efficiency
AI-powered tools can streamline administrative tasks, such as data entry and appointment scheduling, allowing mental health professionals to focus more on patient care. By automating these processes, healthcare providers can allocate more time to therapeutic interactions. Learn more about efficiency in healthcare at Health Affairs.
3. Real-time Monitoring
Generative AI can facilitate real-time monitoring of patients’ mental health by analyzing data from wearables, mobile apps, and other digital tools. This continuous monitoring allows clinicians to track changes in mood and behavior, making timely adjustments to treatment plans as needed.
For insights into real-time monitoring technology, visit Gartner.
4. Cost-effectiveness
Integrating generative AI into mental health assessment can lead to cost savings for healthcare systems by reducing the burden on human resources and improving the efficiency of care delivery. This cost-effectiveness can ultimately make mental health services more sustainable and accessible. More on cost-effectiveness in mental health can be found at National Institute of Mental Health.
Challenges and Ethical Considerations
While generative AI holds significant promise for mental health assessment, there are several challenges and ethical considerations that must be addressed:
1. Data Privacy and Security
The use of AI in mental health assessment raises concerns about data privacy and security. Ensuring that sensitive patient information is protected from unauthorized access is paramount. Mental health professionals and organizations must implement robust data security measures to safeguard patient data. For more information on data privacy, refer to Electronic Frontier Foundation.
2. Algorithmic Bias
Generative AI models are only as good as the data they are trained on. If the training data is biased or unrepresentative, the AI’s outputs may reflect those biases, leading to inaccurate assessments. It is essential to ensure that diverse and representative datasets are used to train AI models in mental health. Learn more about algorithmic bias from AI Now Institute.
3. Dependence on Technology
As AI tools become more prevalent in mental health assessment, there is a risk of over-reliance on technology at the expense of human judgment. While generative AI can provide valuable insights, it should complement—not replace—the expertise of mental health professionals. To read more on this topic, visit National Center for Biotechnology Information.
4. Ethical Use of AI
The ethical use of AI in mental health assessment raises questions about consent, accountability, and transparency. Patients must be informed about how their data will be used and have the option to opt-out of AI-driven assessments. Additionally, clear accountability measures must be established to address any potential harm caused by AI-generated recommendations. Explore ethical considerations in AI at AI Ethics Lab.
Future Directions for Generative AI in Mental Health
As generative AI continues to evolve, its potential applications in mental health assessment are likely to expand. Future developments may include:
1. Advanced Natural Language Processing (NLP)
Advancements in NLP can enhance the ability of AI to understand and interpret human language more accurately. This capability can improve the assessment of mental health through more nuanced analyses of patients’ communication styles and emotional expressions. For insights into NLP advancements, visit Stanford NLP Group.
2. Integration with Telehealth Services
The integration of generative AI with telehealth platforms can provide more comprehensive mental health care. Combining AI-driven assessments with remote therapy sessions can enhance the effectiveness of treatment by offering real-time insights into patients’ mental health status. For more on telehealth, visit American Telemedicine Association.
3. Collaborative Tools for Clinicians
Generative AI can serve as a collaborative tool for mental health professionals, providing data-driven insights that enhance clinical decision-making. By offering recommendations based on extensive datasets, AI can empower clinicians to make informed choices tailored to individual patients. Learn more about collaborative tools at Psychiatric Services.
4. Continued Research and Validation
Ongoing research is crucial to validate the effectiveness and reliability of generative AI in mental health assessment. Studies should focus on evaluating the accuracy of AI-generated insights and their impact on patient outcomes. For more information on mental health research, visit American Psychiatric Association.
Conclusion
Generative AI has the potential to revolutionize the field of mental health assessment by providing deeper insights, improving efficiency, and enhancing accessibility to care. As technology continues to advance, the integration of generative AI into mental health practices can lead to more personalized and effective treatment approaches.
However, it is essential to navigate the challenges and ethical considerations associated with AI to ensure its responsible and beneficial use in mental health. By fostering collaboration between technology developers and mental health professionals, we can harness the power of generative AI to unlock insights that significantly improve mental health outcomes.
In summary, understanding generative AI’s role in mental health assessment is crucial for mental health professionals and society at large. Embracing this technology can lead to a more proactive approach to mental health care, ultimately improving the well-being of individuals and communities.
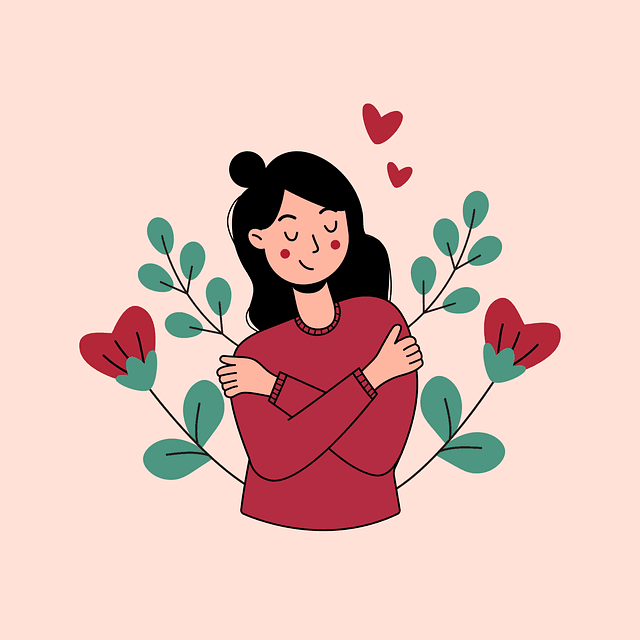